Synthetic aperture radar (SAR) is a class of significantly important remote sensors that work effectively during all weather conditions and all times of day. SAR has the capability to provide very-high-resolution images and multi-dimensional data during limited periods of time, enhancing the spatial-time resolution of observations. In recent years, SAR technology has been developing towards the trend of multi-dimensional imaging and fine-grained image recognition. Meanwhile, the paradigms of SAR imaging and information perception have also been greatly changed to multi-mode, multi-dimensional and intelligent processing strategies. Recently, machine learning and deep learning methods have been applied to SAR imaging and target recolonization to drive various algorithms, which can be classified as model-based and data-learning techniques. Compared to model-based approaches, learning algorithms are more adaptive and show superior performance. However, when limited to small data sets, complex scenes, etc., these learning algorithms may suffer from bad generalization capability and low feature robustness. This Special Issue introduces some newly advanced signal processing and target recognition technologies in SAR, including some new theories, models, concepts and architecture designs for multi-mode/multi-dimensional SAR imaging and parameter inversion, sparse techniques of SAR and ISAR imaging, SAR interference and anti-interference, SAR/InSAR image enhancement, SAR target detection and recognition, SAR image classification and interpretation.
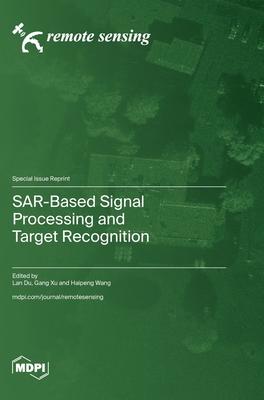
SAR-Based Signal Processing and Target Recognition
Synthetic aperture radar (SAR) is a class of significantly important remote sensors that work effectively during all weather conditions and all times of day. SAR has the capability to provide very-high-resolution images and multi-dimensional data during limited periods of time, enhancing the spatial-time resolution of observations. In recent years, SAR technology has been developing towards the trend of multi-dimensional imaging and fine-grained image recognition. Meanwhile, the paradigms of SAR imaging and information perception have also been greatly changed to multi-mode, multi-dimensional and intelligent processing strategies. Recently, machine learning and deep learning methods have been applied to SAR imaging and target recolonization to drive various algorithms, which can be classified as model-based and data-learning techniques. Compared to model-based approaches, learning algorithms are more adaptive and show superior performance. However, when limited to small data sets, complex scenes, etc., these learning algorithms may suffer from bad generalization capability and low feature robustness. This Special Issue introduces some newly advanced signal processing and target recognition technologies in SAR, including some new theories, models, concepts and architecture designs for multi-mode/multi-dimensional SAR imaging and parameter inversion, sparse techniques of SAR and ISAR imaging, SAR interference and anti-interference, SAR/InSAR image enhancement, SAR target detection and recognition, SAR image classification and interpretation.